Improve In-Year Performance by Addressing Encounter Data Quality Issues
From encounter to regulatory submission, the flow of health data through various systems and organizations creates many potential areas of data degradation, resulting in data quality issues that negatively impact revenue. These issues can lead to as much as 1%-3% of premium lost due to unreported risk, which requires a holistic effort to identify and resolve data quality issues.
For many health plans and provider organizations, the data integrity focus has been primarily on addressing errors identified in government response files, narrowing resolution to only a small portion of the data process. This has been especially significant in the Medicare Advantage (MA) market, where healthcare organizations have invested substantial time and effort to address the reporting migration from the Risk Adjustment Processing System (RAPS) to the Encounter Data System (EDS), as well as to understand the financial impact of risk adjustment factor (RAF) score variances between the two systems. A definite challenge, amplified by the recent Final Call Letter announcement from CMS that accelerates the transition to EDS submissions by increasing the proportion of risk scores derived from EDS from 25% to 50%.
60%-85% of data quality issues exist prior to submission
Pareto’s experience across all regulated markets (MA, Affordable Care Act [ACA], and Medicaid) has uncovered a common theme—that on average 60%-85% of data quality issues exist prior to submission. Though analyzing and understanding rejected submissions and the variances between submissions and responses is a necessary effort, achieving accurate risk scores begins well before regulatory data submissions.
Understanding the Data Lifecycle
Capturing lost revenue due to data quality issues begins at the encounter, ensuring data completeness and accuracy through to regulatory submission (e.g., RAPS/EDS for MA, EDGE for ACA). This requires a detailed understanding of the complex web of systems and data transfers, and the continual updates that take place through the process.
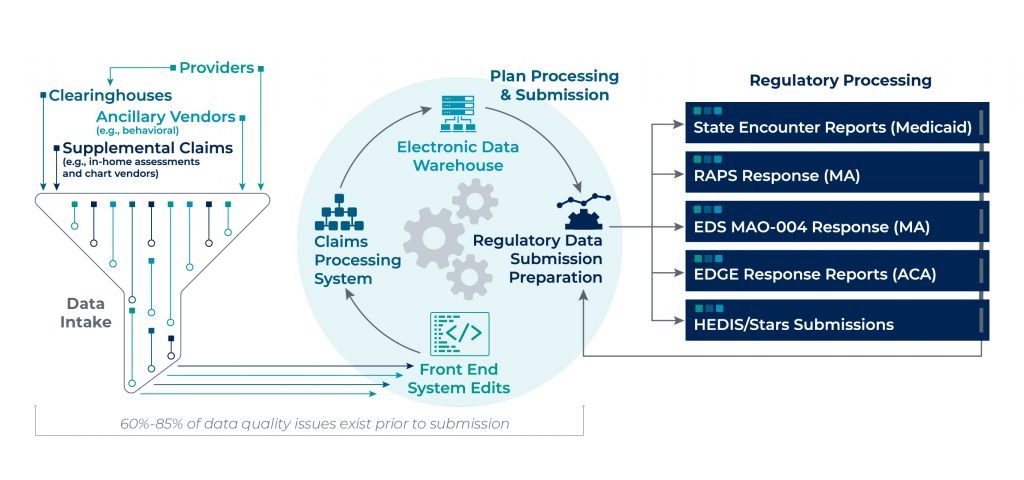
There are various points of potential data leakage along the encounter process and a myriad of root causes to watch out for, which can cause discrepancies that lead to inaccurate risk scores:
- Diagnosis Truncation: Electronic Health Record (EHR) or clearinghouse system truncating evaluated and documented conditions, resulting in incomplete RAPS/EDS or EDGE submissions.
- Vendor Collection: Acquiring timely and complete information from all relevant partners that impact risk scores.
- Unresolved Errors: Not correcting and resubmitting error records.
- Matching Back to Encounter: Inability to tie supplemental chart review records to their corresponding encounter for accurate add/delete determination and submission to CMS.
- Data Transformation: Continued updates and modifications to claims intake and processing systems have negative downstream impacts on the critical data elements needed for reporting.
- Timing of Government Submissions: Claim and/or enrollment information not available for submission by regulatory deadlines; for example, ACA claims that are paid after 4/30.
- Incorrect Claims on 837 Files: Inaccurately coding claims (e.g., service code, diagnosis code), either over- or under-coding.
- Inappropriate Submission Filtering: Not applying the most accurate and current filters when making submission determinations.
- Missing Claims and/or Membership: Data leakage during the transmission of data from one system to the next.
- Duplicate or Inconsistent Claim, Member or Policy Information: System errors causing duplication or manipulation of critical data required for submission acceptance.
Implications of Unresolved Data Quality Issues
Due to the complex nature of the end-to-end encounter process, and how often data “changes hands,” it’s no surprise that many institutions face issues in their data quality. Gone unresolved, these issues can lead to a number of future consequences:
- Lower Financial Reimbursement: Our experience from evaluating the encounter data quality of over 5 million members across MA, ACA and Medicaid has found that, on average, data quality issues lead to $30 to $50 per member per year (PMPY) impact on overall premiums.
- Greater Compliance Risk: Unsupported risk submissions can significantly impact audits (e.g., RADV), as well as pose compliance risk as regulatory agencies are placing greater onus on payers to ensure the accuracy of their submitted documentation.
- Incorrect Member Insights: Data degradation impacts insight into the complete clinical profile of each member, potentially resulting in the mis-prioritization of clinical, cost and revenue management initiatives. As health plans and providers invest in data analytics to further clinical care management, those insights are only as valuable as the completeness and accuracy of information being analyzed.
What Can Healthcare Organizations Do?
Considering the many possible points of failure and the implications of leaving those issues unresolved, healthcare organizations might wonder what steps should be taken to resolve data quality issues.
Evaluate Current Data Processes
The first step is to understand the overall data process at your organization and the interdependencies across providers, clearinghouses, vendors, processing systems, and human capital. Performing a high-level diagnostic of risk score differences between internal claims, regulatory response files and industry benchmarks provides initial insight into potential exposure.
Determine Data Availability & Prioritize Efforts
Assessing data quality from the clinical record or clearinghouse may be a challenge due to limited access to information. Also, for providers, gaining access to data that exists within payer systems can be similarly challenging. Begin by evaluating key areas of the process that present the most potential risk yet require the least effort to resolve based on a qualitative assessment (the “low-hanging fruit”).
Quantify Current Exposure
In order to gain the most out of your investment, it’s important to perform a detailed “data trace” across all available data sources. This process can be a comprehensive review of all data or a focused review on a statistically validated sample, but the review should include a risk score recalculation and an assessment of critical data elements (e.g., provider taxonomy, procedure codes, bill type codes) that are required for submission. The results can be used to prioritize efforts on the issues with the highest potential value from remediation.
Determine Root Cause & Remediate Issues
Once issues have been identified, take the evaluation a step further to determine the root cause. You will need to work with applicable resources (e.g., vendors/partners, IT, business personnel) to validate and resolve issues at the source and prevent recurrence.
Incorporate Ongoing Controls
As systems update, business requirements evolve and government regulations change, so does the data required for submission and what elements are important to ensure your processed risk scores reflect the information you have in-house. This requires ongoing monitoring throughout the year to proactively resolve issues before regulatory submission deadlines.
Maximize the PY2020 Submission Deadline Extension & Improve RAF Accuracy
With CMS’ extension of the PY2020 submission deadline, now is the time to capitalize on this extension and perform an end-to-end encounter data quality assessment to improve risk score accuracy.
Pareto's data quality assessment is designed to recover lost data, improve processes by reducing errors, and identify instances of incorrectly captured and submitted encounter information that pose a compliance risk to help you achieve complete and accurate encounter submissions and improve risk score accuracy.